Yihua Zhang
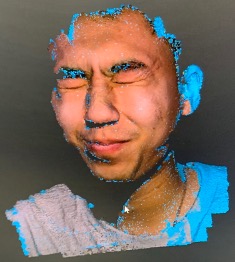
Room 3210
428 S Shaw LN
East Lansing, Michigan
United States of America
Yihua Zhang (张逸骅) is a fourth-year Ph.D. student at OPTML Group at Michigan State University, under the supervision of Prof. Sijia Liu. His research centers on trustworthy and scalable machine learning (ML) algorithms for large language models (LLMs) and diffusion models (DMs), with a keen focus on bridging theoretical foundations and real-world applications. In recognition of his outstanding contributions, Yihua was honored with the IBM PhD Fellowship 2024, the CPAL 2025 Risiting Star Award, and the prestigious MLCommons Rising Star Award in 2024. Yihua has gained valuable industry experience through internships at leading technology companies such as Meta AI, Amazon AWS AI Lab, and Cisco Research. Yihua’s work is driven by the need to develop efficient, scalable, and robust ML algorithms, with a commitment to addressing modern challenges in these domains.
Research Keywords: Machine Unlearning, Jailbreak Attack, Adversarial Training, Fairness, Parameter-Efficient Fine-Tuning, Memory-Efficient Fine-Tuning, Mixture-of-Experts, Model Sparsity, Large Language Model, Diffusion Model, Bi-Level Optimization, Zeroth-Order Optimization.
Theme 1: Trustworthy Foundation Models: Robustness, Fairness, and Unlearning: Yihua explores how to enhance the trustworthiness of foundation models, focusing on robustness against adversarial attacks, fairness in decision-making, and the emerging area of machine unlearning to ensure data privacy and compliance with deletion requests.
Theme 2: Scalable Foundation Models: Efficient Models, Data, and Algorithms: In this theme, Yihua’s work revolves around designing models that are not only powerful but also computationally efficient. His research includes advancements in model sparsification, memory-efficient fine-tuning techniques, and optimizing data usage for large-scale models.
Theme 3: Optimization in Modern ML: Bi-Level and Zeroth-Order Optimization
This research line focuses on the theoretical underpinnings of scalable machine learning algorithms, addressing real-world constraints through bi-level optimization and zeroth-order optimization.
Collaboration Opportunities
I am always open to collaborations with researchers, as well as undergraduate and graduate students seeking Ph.D. positions. While my primary research focuses on trustworthy and scalable ML algorithms for LLMs and DMs, I am also interested in exploring a wide range of topics beyond these areas. If you have exciting research ideas or are looking for opportunities to conduct research under professional guidance, feel free to reach out to me. Please refer to my collaboration statement for more details. You are also welcome to befriend me on Wechat or connect me through LinkedIn.
News
May 16, 2025 |
![]() |
---|---|
May 1, 2025 |
![]() |
Apr 16, 2025 |
![]() |
Feb 26, 2025 |
![]() |
Jan 22, 2025 |
![]() |
Jan 21, 2025 |
![]() |
Jan 20, 2025 |
![]() |
Jan 15, 2025 |
![]() |
Jan 10, 2025 |
![]() |
Dec 15, 2024 |
![]() |